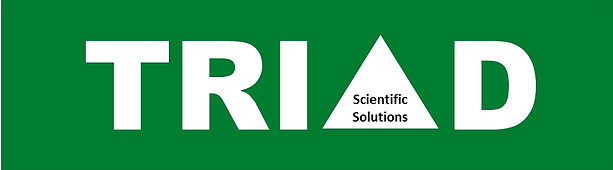
Spring 2017 - Current Topic
FINDING THE GOOD IN GOOD SCIENCE
Note: This document expresses Dr. Jenke’s personal views and opinions and is not a position established and supported by Triad Scientific Solutions, LLC. This document is not professional advice and does not constitute a professional service provided by either Dr. Jenke or Triad Scientific Solutions.
When members of the E&L community gather to develop standards, guidelines and best demonstrated practice recommendations, there are three principles they should obey:
-
The standards must be based on “good science”,
-
The standards must be effective and efficient, and
-
The standards must fit every conceivable circumstance well.
It is the inability to achieve these principles that makes the generation of standards, guidelines and recommendations so frustratingly challenging.
Consider the last principle, for example. It is intuitively obvious in a diverse field such as pharmaceuticals that this principle is impossible to achieve as it is clear that a rigorous standard (which is specified set of tests coupled to a specified set of acceptable test results) cannot fit all the diverse circumstances equally well. It is the same problem as trying to design a glove that fits every human being. If the underlying purpose of the glove is “to keep one’s hands warm”, then a standardized glove can be designed that will address this requirement, to some degree, for most people. However, because the glove must keep everybody’s hands warm, it is logical that there will be design tradeoffs which will mean that while it keeps everyone’s hands warm, it does not keep everyone’s hands as warm as they would personally like it. Furthermore, there may be other trade-offs, such as “these gloves are not very sexy”, or “these gloves do not match my coat” or “these gloves make my hands itch”.
While the challenges in making standards that are generally applicable in the greatest number of circumstances are considerable, this is not the point that I want to address in this discussion. Rather, I want to address the challenges associated with good science.
Good science suffers from with the same problem as designing a standardized glove. As good scientists, we learned and we understand that there are very few universal scientific truths; rather, a scientific truth is a truth only under the rigorously defined set of circumstances upon which it is based. When we perform an experiment and draw a conclusion from that experiment, we understand the conclusion is only perfectly valid for the set of defined experimental circumstances we started out with. Extension of that same conclusion to other circumstances involves a certain measure of risk, specifically the risk that in changing the circumstances we have invalidated (knowingly or unknowingly) some fundamental principle that defines the applicability of our conclusions. Thus, we understand that there is an inherent trade-off when we make scientific generalizations and put them into standards, guidances and recommendations. That is, we sacrifice some of the good in good science for the sake of providing a direction that is generally right in the greatest number of circumstances.
The challenge we face as practitioners of good science is not in recognizing good science per say but recognizing the boundaries that differentiate between good science properly applied and good science improperly applied. When we are tempted to use a standard, or leverage a “rule of thumb” or “do this because everybody else is doing it”, as good scientists we must ask ourselves “am I taking a good idea in certain circumstances and applying it to the wrong circumstances?”. If the answer is yes, then surely this is as bad as using “bad” science in the first place.
Let me illustrate this with an example. I use this as an example not because it a particularly bad practice but because it effectively illustrates my point. The following, taken from the PQRI OINDP Best Practice recommendations, is well known and commonly applied in the E&L community.
-
The Working Group recommends that analytical uncertainty be evaluated in order to establish a Final AET for any technique/method used for detecting and identifying unknown extractables/leachables.
-
The Working Group proposes and recommends that analytical uncertainty in the estimated AET be defined as one (1) standard deviation in an appropriately constituted and acquired Response Factor database OR a factor of 50% of the estimated AET, whichever is greater.
The question I would ask you to consider is “where is the good science and the not so good science in these recommendations?”
Here is my answer. It is well known that response factors will vary among the universe of compounds that could be extractables and leachables. Thus, it is good science that a general concept such as the AET, which presumably is applicable to all possible extractables/leachables, take this variation into account. Furthermore, we all understand that basing actions on relevant and sufficient data is the cornerstone of good science, and thus that the requirement to consider “an appropriately constituted and acquired Response Factor database” is a requirement to do good science. However, it must be obvious that the direction to universally “use a factor of 50%” is not necessarily good science. While the derivation of the 50% is itself good science, as it was based on a response factor database (which is somewhat small in the context of the databases available today), it is obvious that the 50% is only relevant for the compounds in that database and the analytical method with which the data was generated. Universal and unquestioned application of the factor of 2 rule to compounds that were not in the original database and to analytical methods other than the method used to generate the data is not the best science; rather, it is poor science, not because the science itself is bad but because the good science aspects are being applied out of context.
To a good scientist, arguments such as “it is better than nothing” or “everybody else is doing it” are inexcusable. Certainly, the idea that “it is better than nothing” has to be examined objectively and harshly. The improper application of science is not guaranteed to be better than doing nothing because it is not the case that the improper application of science will always make things better. In fact, the history of improper application of good science is littered with examples of bad outcomes derived from applying good sciences incorrectly.
Listen, nobody said doing good science was easy. We understand that part of the driving force for recommending that the factor of 2 be universally applied is that back then few people could access a database. Thus, it was nearly impossible to practice the good science required in the recommendation and people, rather than do nothing, gravitated to the other part of the recommendation. However, today, it is virtually impossible to run into a reputable E&L laboratory that is not eager to talk about their database. Thus, in this case, our ability to do good science has finally caught up with our responsibility to do good science. It is proper that we accept that responsibility and be held accountable for meeting that responsibility.
This is true not only to adjusting the AET for analytical uncertainty but in numerous other places where our current capabilities enable our ability and address our responsibility to practice and preach a higher degree of good science than has ever been possible. Currently applied recommendations, standards, guidelines and practices must be adjusted, as appropriate, to leverage this new and higher degree of good science and new recommendations, standards and guidelines must be drafted to reflect this new and higher degree of science. We aspire to better science because we are capable of better science. More importantly, if we are going to talk the talk, we best start walking the walk.
Triad Scientific Solutions' mission is to provide the pharmaceutical, cosmetic, food and related industries with integrated, science-based, and practical solutions to suitability for use challenges for packaging, manufacturing components and systems, and administration devices.